Now that we’ve explore how genAI can be used as a tool for OER, there is a greater question: should it? Let’s explore some of the ethical issues in using generative AI for OER.
Copyright
“Because we trained the machines. All of us. But we never gave our consent. They fed on humanity’s collective ingenuity, inspiration and revelations (along with our more venal traits). These models are enclosure and appropriation machines, devouring and privatizing our individual lives as well as our collective intellectual and artistic inheritances.” -Naomi Klein, The Big Bot Problem
There are multiple areas where copyright should be considered when using genAI; two important areas to consider when using it for OER are the content that the genAI tool has been trained with and the content that the genAI tool generates:
- Use of content to train AI models: Many generative AI tools have been trained on copyrighted works often without the permission of copyright holders. While some organizations may argue that this use is considered fair dealing under current copyright legislation, there are a number of lawsuits where creators are arguing that GenAI tools are creating unauthorized derivatives and have stolen or appropriated their work. This also means that AI-generated content could be subject to future copyright claims.
- Applying copyright to generated output: Can work created by AI be copyrighted? This question remains uncertain, as copyright law is often shaped by court cases and legal challenges. In most jurisdictions, including Canada, copyright law generally requires human authorship—works must be “original” and reflect human skill and judgment to qualify for protection. As a result, purely AI-generated content typically cannot be copyrighted. However, if a human significantly contributes to the creation process—for instance, by crafting detailed prompts, editing output, or making substantial modifications—their contribution may be eligible for some copyright protection, even if the AI-generated portion itself remains uncopyrightable. In recognition of these complexities, the Canadian government launched a public consultation in October 2023 titled “Copyright in the Age of Generative Artificial Intelligence” to explore how current laws should adapt, though no legislative changes have been made yet. Adding further complexity, some AI platforms like OpenAI or Midjourney have varying terms of service that influence ownership and licensing of AI-generated works.
Veracity and Accuracy

GenAI tools are well known to produce inaccurate or made-up answers, which are referred to as hallucinations. This flowchart at the right outlines the process of using for considering if you should use genAI, in this case ChatGPT, for your task. The key questions it suggests are:
- Does it matter if the output is true
- If it does, do you have the expertise to verify if the output is accurate?
- Are you willing to take responsibility for missed inaccuracies
Educators and learners need to trust OER to rely on them. If AI-generated content is found to contain errors, it may cause users to question the overall reliability of OER, undermining years of advocacy around their legitimacy and quality. Additionally, if genAI tools generate inaccurate or misleading content, and that content is incorporated into OER without careful review, it can perpetuate false information across educational ecosystems. This is particularly problematic in fields like science, history, or healthcare, where factual accuracy is critical.
Privacy
Privacy is another area of concern when using genAI tools. When instructors or students interact with genAI platforms—especially those hosted by third parties—they may input sensitive data such as names, institutional affiliations, learning needs, or performance information. If this data is not adequately protected, it could be exposed or misused. GenAI tools also often retain user inputs to improve their models. If educators include proprietary content, unpublished research, or confidential institutional materials while generating OER, there’s a risk this information could be stored or later surfaced in other users’ prompts. OER creators should avoid including sensitive or non-public information when using genAI to draft or revise open resources.
The use of genAI in OER creation can also raise concerns about the provenance of content—where it came from and whether it may have inadvertently included private or copyrighted information through training data or prompt context. OER creators should document how genAI was used in the resource development process and ensure proper attribution or disclaimers, supporting transparency and trustworthiness.
Some GenAI platforms track user behavior or prompt history to personalize interactions. While this can improve user experience, it may also lead to surveillance or profiling, particularly harmful in open educational contexts where equity and freedom of thought are valued
Bias
Bias is another important concern that affects the effectiveness and inclusivity of using genAI as a tool for OER. GenAI models are trained on vast datasets that often reflect dominant cultural, linguistic, or ideological norms. These data sets may prioritize certain data sources that may not be open or trustworthy and genAI tools often perform better in English and major global languages, while less widely spoken or regional languages may be underrepresented or poorly handled. When genAI tools are used to generate textbooks, lesson plans, or quizzes, they might:
- Marginalize non-Western perspectives or underrepresented voices.
- Reinforce stereotypes related to race, gender, ability, religion, or geography.
- Omit important contributions from diverse communities in various fields of study and neglect alternative epistemologies (e.g., Indigenous knowledge systems).
- Generate inappropriate, offensive, or factually incorrect outputs.
OER created with biased GenAI may inadvertently perpetuate narrow worldviews, making them less inclusive and less effective for global or diverse learners. Such biases can shape learners’ understanding in ways that ignore pluralistic or interdisciplinary approaches, limiting the pedagogical value of the OER and hindering the democratization of knowledge and access that is central to the OER movement.

Reflection
Try this out: Ask a genAI tool of your choice to create an image of a professor at a university. What doe the genAI created professor look like? What are their surroundings? How does the image reflect the biases inherit in the genAI tool and it’s training data? How could you modify your prompts to overcome such biases?
Equity
While genAI has the potential to make it easier for more people to create content and access knowledge, if it is not introduced in an inclusive and thoughtful way, it could actually make existing inequalities worse.
The most advanced genAI tools are often expensive and locked behind paywalls. Not all instructors or students will have access to the same tools nor the same skills to work with them. Using these tools effectively often requires fast internet, modern devices, technical prowess, and strong computing power—resources that aren’t available to everyone. This creates a gap between those who can take full advantage of genAI and those who can’t. On a larger scale, most companies and countries—especially those in under-resourced regions like the Global South—don’t have the infrastructure or funding to create or control genAI companies or technologies themselves. This limits their ability to shape how these tools are developed and used.Because of this digital divide, students and communities with fewer resources may struggle to use genAI to create or adapt OER. As a result, fewer voices and perspectives may be represented in openly shared content, reducing its diversity and relevance.
There is growing concern that genAI could negatively impact jobs in education, especially as some institutions consider replacing teaching assistants with AI tools to cut costs. While AI can handle tasks like grading or answering basic questions, it lacks the empathy, cultural awareness, and personalized support that human educators provide. In well-funded schools, AI may be used to supplement human help, but in under-resourced institutions, it risks becoming a full replacement—potentially widening the gap in educational quality. This could deepen existing inequities, leaving students in underserved communities with fewer meaningful learning supports, while also reducing opportunities for early-career educators.
Environmental Impacts
Environmental concerns should also be taken into consideration when using genAI to create or modify OER. Training and operating genAI models—especially large language models—require substantial computational power, leading to high energy use and carbon emissions. These immense computational resources also generate heat. To prevent overheating and maintain optimal performance, data centers use cooling systems which consume large amounts of water. It is estimated that a single genAI prompt that creates a 500 words of content uses the energy equivalent to charging a smartphone for 5 minutes and consumes a standard size bottle of water.
OER advocates often focus on ethical and sustainable practices. Individuals can also chose to run genAI models on their own computers which can reduce the load on large data centers and avoid water-intensive cooling systems. They can also use smaller genAI models which consume far less energy and water than large models like GPT-4. These models are often great tools for core OER activities such as creating quizzes, lessons, or adapting content.
It’s also important to recognize that universities and institutions have significant leverage when selecting technology vendors. When partnering with genAI companies, Universities should prioritize platforms that are transparent about their energy use, water consumption, and carbon emissions, and that actively pursue sustainability goals. Being aware of these often-hidden resource costs enables educators and institutions to make more informed, responsible decisions.
Recommendations and Guidelines
It’s important to use genAI thoughtfully. The following list, which was partially adapted from BCcampus, provides some guidelines and recommendations for using gen AI for OER:
- Be cautious with your use of AI generated content. Use genAI for tasks where it adds real value—not just for novelty.
- Manually review and assess all AI generated content for accuracy, appropriateness, and usefulness before including it in any OER. AI generated content should be reviewed by more than one subject matter expert to ensure the validity of the content. As an OER author, you are ultimately accountable for the content that you share in your OER, therefore you must manually verify the accuracy of the content.
- Closely review any AI generated content for bias, including language or images that reinforce cultural or societal stereotypes around race, ethnicity, colour, ancestry, place of origin, political beliefs, religion, marital status, family status, ability, sex, gender identity and expression, sexual orientation, age, and class and/or socioeconomic status. Consider reviewing and assessing the outputs of AI generated content using your institution’s EDI statements and ask whether the content aligns with these considerations.
- Do not use generative AI to generate content for an area or subject where you do not have the appropriate level of knowledge or understanding to verify the accuracy of the content. f you use AI to create a summary of another work, you should ensure that you are familiar enough with the original work to determine whether or not the generated summary is an accurate representation of the original work before using the summary.
- Be transparent about your use of generative AI. Just like attributing the reuse of open content, you should include statements within the OER that let others know that you have used generative AI in the creation of the OER. This should include;
- what content was generated
- what tools were used to generate the content, including links to the tool,
- how you used that tool (ie what prompts was the tool given that generated the content)
- the date the content was generated
- what steps were taken to review the content to ensure it was valid and correct.
- Be cautious with intellectual property
- As much of the legal consensus around AI generated content suggests AI created content is not copyrightable, you should not apply a Creative Commons license to AI generated content as Creative Commons licenses can only be applied to content that is copyrightable.
- Ensure accurate representation: prompt genAI to reflect diverse viewpoints and challenge dominant narratives. Use or advocate for GenAI models trained on inclusive and representative datasets to minimize cultural, linguistic, and demographic bias.
- Support open-source, community-driven AI models that are smaller and more efficient. Advocate for transparency and sustainable tools at the institutional level.
Adapted from OER Publishing at BCcampus: Generative Artificial Intelligence Guidelines and Recommendations under a CC BY 4.0 license.
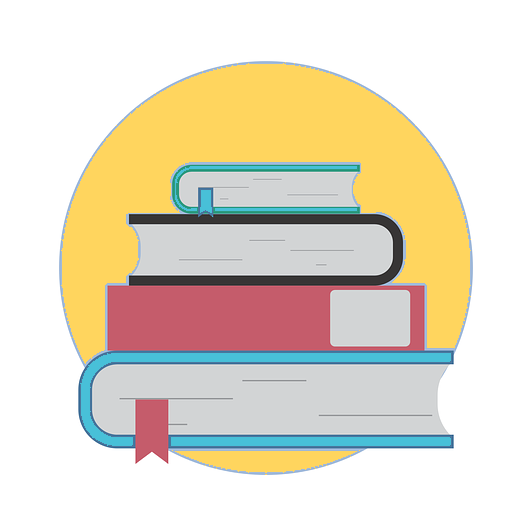
Dig Deeper
To learn more about genAI and OER, review:
- Flexible Learning for Open Education (FLOE) Project — AI in Education: A collection of articles from leading educators and researchers, supported by the William and Flora Hewlett Foundation in partnership with Etika Insights.
- The Psychosocial Impacts of Generative AI Harms – study by Vassel et al (2024) that explores the experiences of users with marginalized and minoritized identities emphasizing the need for a critical understanding of the psychosocial impacts of genAI tools when deployed and utilized in diverse social contexts.
- Judicious AI Use to Improve Existing OER – an article by Kimmons et al (2025) that explores in part how open education can serve as a space for more sustainable and equitable uses of AI to achieve socially valuable goals.
- Navigating AI in Open and Higher Education: Critical Guidelines and Practical Applications by Maja Bali, Equity Unbound / American University Cairo
- The AI + Open Education Initiative from MIT Open Learning is a series of rapid response papers and multimedia projects about how genAI might accelerate or hinder the promise of open education.
- BCcampus GenAI in Teaching and Learning Toolkit: The Least You Need To Know as Educators